5 Best Practices for Building an AI Predictive Analytics Model
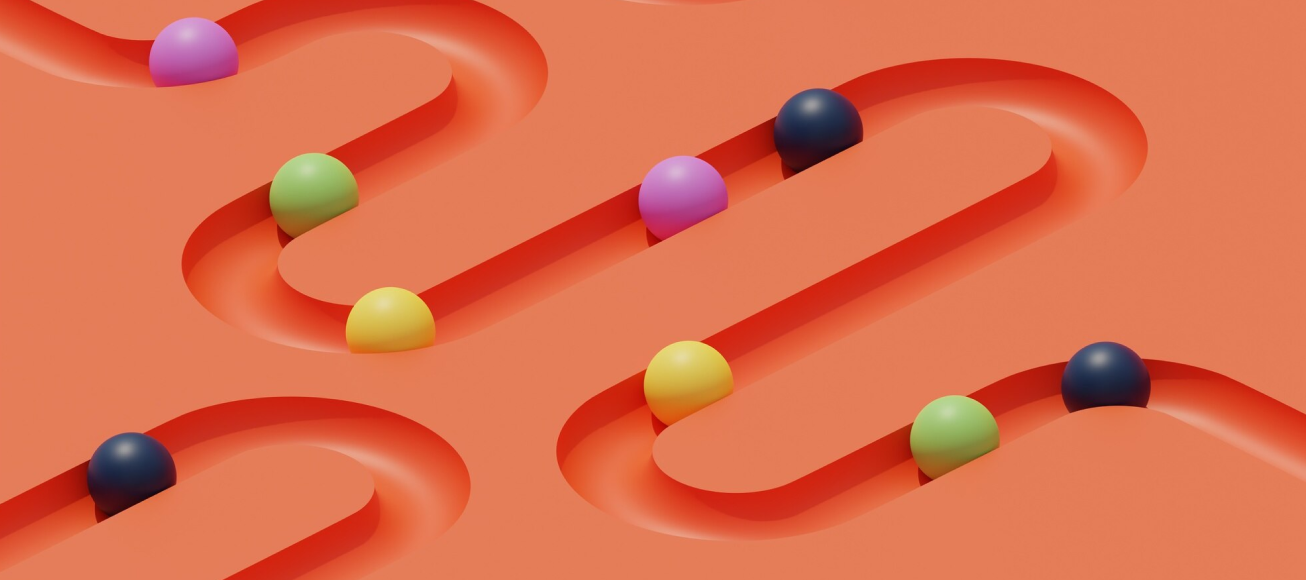
Predictive analytics models powered by AI can transform businesses by identifying trends, optimizing decision-making, and uncovering new revenue opportunities. However, building an effective AI-driven predictive model requires more than just feeding data into an algorithm. A strong foundation, the right data strategy, and continuous refinement are crucial for success.
To maximize accuracy and ensure long-term scalability, organizations must follow key best practices when developing predictive analytics models. Here are five essential steps to guide the process.
1. Define Clear Business Objectives and Success Metrics
The most advanced AI model is useless without a clear purpose. Before building a predictive analytics model, businesses must define specific objectives and establish success metrics that align with their goals.
Start by identifying the problem you want to solve. Are you looking to predict customer churn, forecast demand, optimize pricing, or personalize recommendations? The more precise the goal, the easier it will be to determine which data to collect and how to structure the model.
Once the objective is clear, define key performance indicators (KPIs) that will measure the model’s success. These may include:
- Accuracy and precision of predictions
- Reduction in customer churn after implementing predictive insights
- Increased sales or conversions due to AI-driven recommendations
- Efficiency gains in supply chain or resource allocation
Having well-defined objectives ensures the model remains aligned with business priorities and delivers measurable value.
2. Gather and Prepare High-Quality Data
AI models are only as good as the data they are trained on. Poor-quality, incomplete, or biased data can lead to inaccurate predictions and unreliable insights. Ensuring high-quality data collection and preparation is critical for predictive analytics success.
Best practices for data preparation:
- Prioritize first-party data: Collect data directly from customers through interactions, purchases, and engagement rather than relying on third-party sources.
- Clean and preprocess data: Remove duplicates, handle missing values, and standardize formats to create a clean dataset.
- Ensure diversity and completeness: Include a wide range of data points, such as transactional data, behavioral patterns, and contextual signals, to improve model accuracy.
- Balance historical and real-time data: While historical data helps identify long-term patterns, real-time data improves adaptability to changing market conditions.
A well-structured and diverse dataset lays the groundwork for an AI model that produces meaningful, actionable predictions.
3. Choose the Right AI Model Template
Selecting the right features for an AI model is crucial to its success. Pre-built predictive models can accelerate implementation and provide immediate insights. Some essential AI model templates include:
- Prediction to Purchase: Determines how likely a visitor is to make a purchase by the end of the session.
- Prediction to Abandon Checkout: Identifies the likelihood of checkout abandonment.
- Prediction to Join Rewards Program: Estimates the probability of a visitor enrolling in a loyalty program.
Choosing the appropriate predictive model features enables businesses to tailor user experiences, improve targeting strategies, and enhance decision-making processes.
4. Continuously Train, Test, and Optimize the Model
AI models are not a one-time solution—they require continuous learning and improvement to maintain accuracy and relevance. Once a predictive analytics model is built, it must be rigorously tested, validated, and refined to ensure reliability.
Key optimization techniques:
- Cross-validation: Use multiple training and testing sets to assess model performance and avoid overfitting.
- A/B testing: Compare model-generated insights with baseline business strategies to measure effectiveness.
- Hyperparameter tuning: Adjust model parameters to optimize predictive accuracy.
- Regular retraining: Update the model with new data to adapt to changing patterns and customer behaviors.
By continuously monitoring and refining predictive models, businesses can ensure they remain responsive and aligned with real-world dynamics.
5. Ensure Ethical AI Use and Explainability
With great predictive power comes great responsibility. AI-driven analytics models must be designed with transparency, fairness, and ethical considerations in mind to avoid unintended biases and ensure trustworthiness.
Ethical best practices in predictive AI:
- Bias detection and mitigation: Regularly audit data and model outputs for biases that could lead to unfair outcomes.
- Explainability and transparency: Use interpretable AI techniques to ensure stakeholders understand how predictions are made.
- Compliance with privacy regulations: Adhere to data protection laws like GDPR and CCPA by ensuring ethical data collection and consent-based personalization.
- Customer-centric AI: Use predictive analytics to enhance user experience and create meaningful interactions rather than relying solely on profit-driven strategies.
By prioritizing responsible AI practices, businesses can build trust with customers and stakeholders while ensuring long-term success with predictive analytics.
Unlocking Competitive Advantage with AI-Driven Predictive Analytics
Building a predictive analytics model requires more than technical expertise—it demands a strategic, data-driven approach that prioritizes accuracy, scalability, and ethical responsibility. By defining clear objectives, ensuring high-quality data, selecting the right model, continuously optimizing, and maintaining transparency, businesses can harness AI to unlock powerful insights and drive smarter decision-making.
Organizations that follow these best practices will gain a significant competitive advantage, turning predictive analytics from a technical capability into a true business differentiator. The future belongs to those who can not only predict what’s coming but also take proactive action to stay ahead.