Traditional vs. Adaptive AI
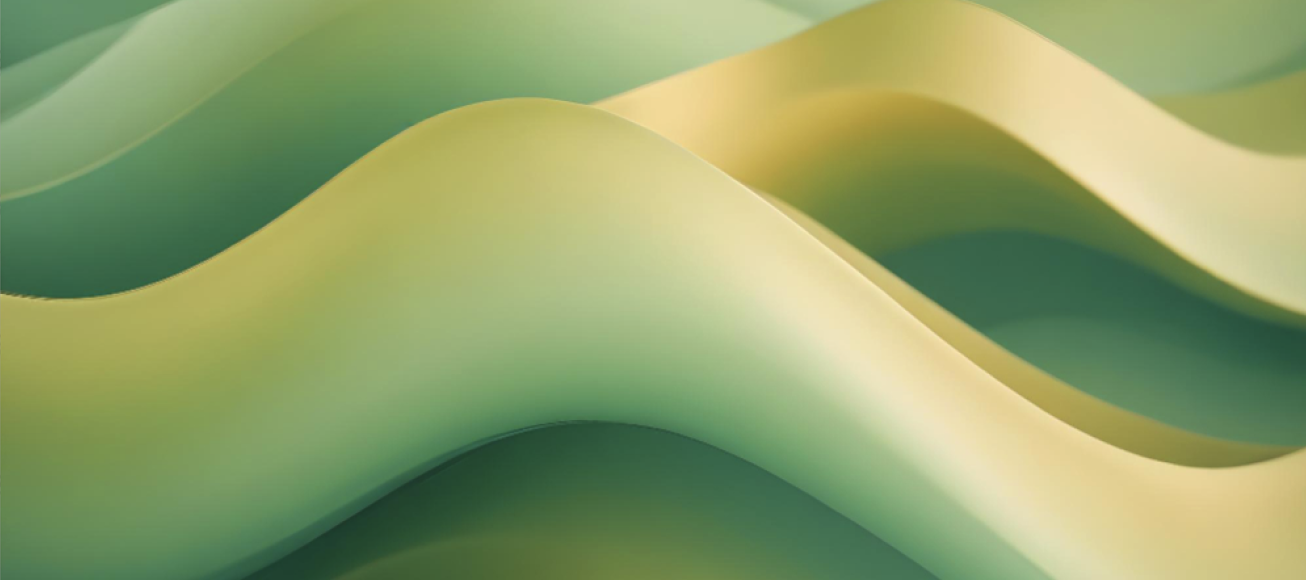
Digital consumer culture and behavior is constantly shifting, and the success of those who provide services online is often determined by their ability to adapt to and preempt these changes. Recently, the advent of AI has caused the most seismic transformation to the digital realm in a decade, resulting in the automation of many previously time-intensive processes.
However, not all AI is created equal. In this article, we’ll discuss the pros and cons of traditional and adaptive AI, and demonstrate how the latter is likely the better fit for your marketing and predictive needs.
What Are Traditional and Adaptive AI?
Traditional AI systems have been around a lot longer than their adaptive counterparts, with the first examples appearing in the 1950s. Built to solve specific pre-programmed tasks, such as search functions, order processing, and shipping logistics, these systems rely on hard coding and extensive training with data sets. Common traditional AI applications include product search functions, managing accommodation and travel reservations, payment fraud detection, and automated customer service chatbots.
The first (rudimentary) adaptive AI systems (also known as machine learning) emerged in the 1980s, but it is only recently that significant technological advancements have seen adaptive AI saturate the public sphere in the form of sophisticated language learning models such as Chat GPT and Claude AI.
Unlike traditional AI, these systems adapt and improve functions on their own based on environmental feedback. Rather than simply following static rules, adaptive AI examines patterns to gain insights that optimize future decisions, predictions, and business outcomes. A few applications of adaptive AI include recommendation engines, dynamic pricing models, personalized promotions, predictive analytics, and even generating interactive gaming characters.
How Does Traditional AI Compare to and Work With Adaptive AI?
The main advantage of traditional AI is efficiency at repetitive administrative and operational tasks, allowing your employees to focus on more creative work. Traditional systems offer reliability and consistency, and can even improve over time, as long as they can remain within their strict, predefined parameters. However, these programs cannot deviate from their assigned tasks and will struggle or fail outright when dealing with unforeseen events or data that falls outside their usual remit.
Adaptive AI compliments the more rudimentary services performed by traditional AI by performing complex tasks that involve adapting swiftly to new and fast-changing circumstances. These advanced systems are trained using a combination of supervised, unsupervised, and reinforcement learning, leading to far greater problem-solving capabilities than traditional models.
While adaptive AI can be used in conjunction with traditional AI, it can also replace almost any trad-AI function, and perform the task with great efficiency. This saves great deals of time that would otherwise be spent manually tuning these systems to cope with new kinds of information. For instance, traditional AI chatbots are programmed to respond to certain questions but fail when presented with anything outside their training. Adaptive AI chatbots continually enhance their language processing from ongoing customer conversations, enabling them to address novel questions and scenarios.
These advantages have not gone unnoticed by software experts. For instance, Gartner expects that in the next three years, enterprises that adopt adaptive AI systems will operationalize artificial intelligence models 25% faster than peers using traditional AI and that by 2025, 30% of new applications will use adaptive AI to power personalized adaptive user interfaces, up from less than 5% today. Let’s look at a few ways that adaptive AI can give you the edge over more traditional competitors, alongside examples of companies and industries that have achieved it:
- Personalization at scale: by processing thousands of data points from the behavior of every user, adaptive AI delivers hyper-personalized recommendations and experiences. Netflix and other streaming platforms leverage adaptive AI to suggest personalized content for each subscriber by analyzing their viewing history and how they rate different shows/movies. This allows recommendations to continuously improve.
- Automated experimentation: adaptive systems test new engagement strategies, promotions, and features based on live data. Platforms like Google Ads and Meta Ads use adaptive AI to automatically test different ad creatives, landing pages, audience segments, bids, and more to optimize campaign performance over time. These platforms enable advertisers to set up “ad experiments”. The adaptive AI will then serve different ad variants to website visitors in real-time and detect the best high-performing combinations based on user engagement and conversion data.
- Competitive response agility: adaptive AI automatically adapts pricing, inventory, creative offers, etc. empowering real-time responses to competitors in fast-changing online sectors. Ride sharing platforms like Uber and Lyft rely on adaptive AI to parse data on competitor behavior as it happens across locations – then instantly match price changes and adjust rider promotions/rewards in real-time. For example, if analysis shows a rival has lowered prices by 10% in a city to gain market share, the AI will automatically adjust pricing to match within minutes to protect its customer base. The AI also tweaks promotional offers like discounted rides, coupons or loyalty perks to entice riders.
- Improved UX and conversions: harnessing machine learning insights about each user, adaptive AI removes friction and optimizes persuasive customer experiences online. Many online retail chains, such as Target, utilize adaptive AI to optimize their customer experience by analyzing the macro shopping patterns of anonymous visitors. Using this data, they can refine store layouts, pricing promotions, and recommendations over time to convert more customers.
- Future forecasting: by detecting new trends and signals, adaptive AI better predicts future outcomes, needs, and challenges allowing online businesses to prepare for the weeks and months ahead. For instance, fintech companies like Robinhood utilize adaptive AI to analyze historical trading data, news trends, social media chatter, and user patterns. This enables them to forecast stock price changes and identify potential market rally moments or months ahead of time.
Adapt to Survive
Evolutionary biologists argue that the ability to adapt gave humans the upper hand throughout the millennia. Now, online retailers are discovering that the adaptability of the AI they use is equally essential for survival. Let’s briefly recap the four key differences between traditional and adaptive AI:
- Learning Ability: Adaptive AI systems can learn dynamically from new data in real time, while traditional AI relies on static data sets for training models.
- Automated Improvement: Adaptive AIs contain in-built feedback loops and algorithms that allow them to enhance their own decision making without human intervention. Traditional AI systems are rule-based, comparatively simple, and cannot update on their own.
- Flexible Scaling: Adaptive AI can scale insights across components as data grows exponentially. Traditional AI generally lacks scalable infrastructure, sometimes leading to breakages.
- Adaptability: The key is in the name – Adaptive AI is designed to handle unfamiliar inputs and scenarios through contextual learning, unlike rule-based systems that fail outside specific parameters. For this reason, adaptive AI is well suited for almost any task that traditional AI is designed for, but the reverse is seldom the case!