What is Adaptive Search and How Does it Work?
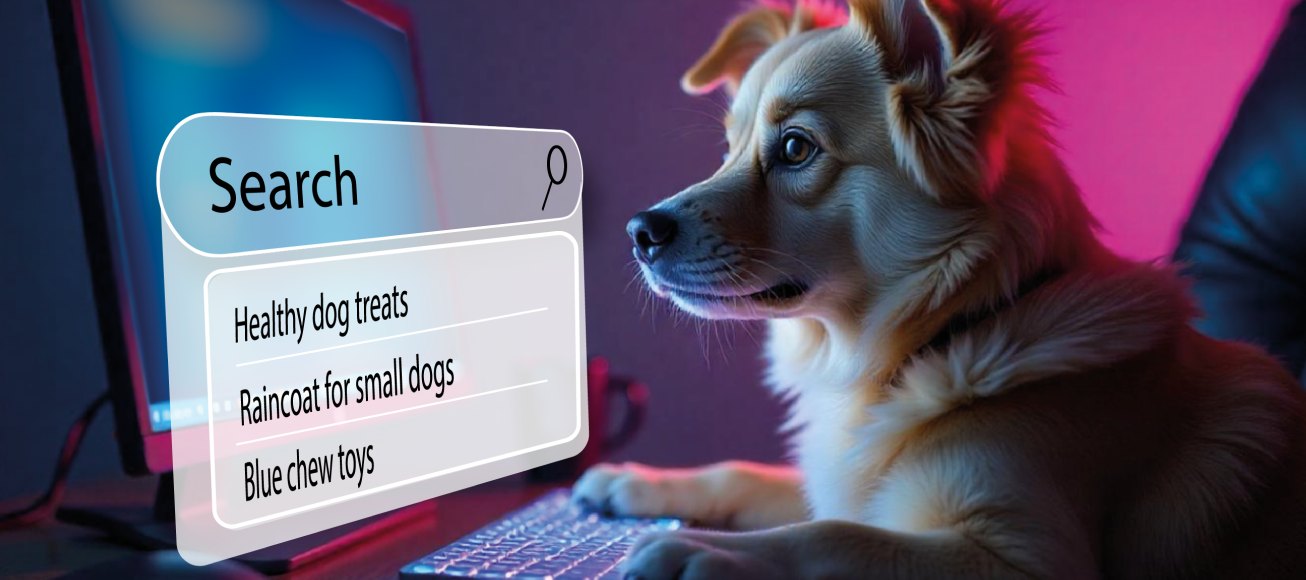
In the e-commerce landscape, where customer attention is limited and expectations are high, ensuring that users find what they’re looking for quickly and efficiently can make or break a business. The search functionality on an e-commerce website is often the first interaction a potential customer has with your catalog, and it plays a critical role in their decision-making process. Enter adaptive search.
In this article, we will explore how adaptive search technology that tailors search results based on user behavior, preferences, and real-time interactions, works in the context of e-commerce. We’ll also discuss how it improves customer experiences and why it’s quickly becoming a standard solution for online retailers aiming to boost conversions and engagement.
Understanding Adaptive Search in E-Commerce
Adaptive search in the e-commerce space refers to a search engine’s ability to adjust and refine results based on a user’s individual preferences, past interactions, and real-time behavior. Unlike traditional e-commerce search engines, which rely on static algorithms and keyword matching, adaptive search learns from every user interaction, using this data to improve future search results.
The goal is to deliver personalized product recommendations, relevant search results, and faster access to desired items, all of which contribute to a more seamless, enjoyable, and successful shopping experience. Adaptive search doesn’t just serve the same list of products for every user typing in similar queries; it tailors the search experience for each and every shopper based on a few areas:
- Browsing history: What items the user has looked at or added to their cart.
- Purchase history: Past purchases can influence future search results.
- User preferences: Whether the user tends to favor certain brands, price ranges, or product categories.
- Device and location: Searches can adapt based on whether the user is on mobile or desktop, or where they’re located geographically.
- Real-time behavior: How users interact with the site during a session, including the amount of time spent on particular pages or which product images they engage with.
The adaptive nature of these search systems means they become more intelligent and effective over time, continuously refining the shopping experience based on ongoing customer behavior and interactions.
How Adaptive Search Works in E-Commerce
Adaptive search in e-commerce combines several advanced technologies, including machine learning (ML), artificial intelligence (AI), and natural language processing (NLP), to dynamically adjust search results. Additionally, it leverages non-personally identifiable information (non-PII) from billions of data points across user journeys to accurately predict customer behavior and next actions. Here’s how it works in practice:
- Capturing User Behavior and Non-PII Data
Adaptive search systems capture vast amounts of non-PII data from each user interaction, such as the products viewed, time spent on pages, click patterns, and navigation paths. This data, while anonymized, forms a critical foundation for predicting future behavior and preferences.
By analyzing billions of these data points across the journeys of countless visitors, the system identifies behavioral patterns and trends. For instance, if a significant percentage of users who browse winter jackets also search for accessories like hats and gloves, the system learns to offer those complementary products to new users who show similar patterns.
Non-PII data allows the system to build accurate user behavior models without invading privacy, relying solely on patterns of interaction to improve personalization.
- Utilizing Predictive Models to Anticipate Next Actions
Machine learning algorithms process vast volumes of behavioral data to create predictive models. These models analyze patterns in how users navigate the website, what they tend to click on, and what products they are likely to view or purchase next.
Using non-PII data from billions of user journeys, machine learning enables adaptive search to predict what product a user might be interested in or what their next action could be. For example, if a user consistently browses premium products in certain categories, the system may prioritize high-end products in search results, understanding that this user values quality over price.
Because the system is trained on an enormous volume of anonymous data, it can accurately predict user intent even for first-time visitors, drawing from patterns seen in other similar user journeys.
- Natural Language Processing (NLP) for Query Understanding
Traditional e-commerce search engines rely heavily on exact keyword matches, which can often lead to irrelevant or frustrating search experiences. Adaptive search uses NLP to understand the context and intent behind a user’s query, not just the specific words they use.
For example, the query “luxury running shoes” might yield better results in an adaptive search system because it understands that “luxury” suggests a preference for premium brands. This insight is derived not just from the query itself but from vast amounts of previous data on how users with similar search behaviors act. By recognizing the broader patterns and trends in how users interact with search results, the adaptive system can deliver more precise and relevant suggestions.
- Personalized Search Results Based on Visitor Journeys
The key advantage of adaptive search in e-commerce is personalization. As the system learns from anonymized visitor journeys, it tailors search results to each user based on previous behaviors seen in similar cases. This personalization does not depend on knowing exactly who the user is but rather on understanding their behavior patterns.
For instance, two customers searching for “running shoes” might receive different results: one might be shown budget-friendly options, while another is shown premium, performance-focused models. These predictions are based on the system’s deep understanding of behavioral patterns across similar customer profiles, gathered from vast datasets of non-PII data.
- Real-Time Adaptation and Continuous Learning
One of the most powerful aspects of adaptive search is its ability to respond and adjust in real-time. As users navigate through an e-commerce site, the system learns from their immediate behavior, adapting search results on the fly.
If a user starts looking at specific brands or product categories, the system can adjust search results in real time, displaying products that better match the user’s current focus. This adaptability is made possible through the continuous analysis of billions of data points from prior user journeys, allowing the system to understand evolving customer needs and predict next actions.
By processing non-PII data across large user groups, adaptive search systems can make informed predictions about what a user is likely to do next, optimizing the shopping experience without compromising privacy.
The Benefits of Adaptive Search for E-Commerce Websites
Adaptive search brings numerous benefits to e-commerce websites, improving both the customer experience and business outcomes.
1. Increased Relevance and Precision
With traditional search engines, users often have to sift through irrelevant results, which can lead to frustration and cart abandonment. Adaptive search ensures that the products displayed are highly relevant to the user, based on their preferences and past interactions. This precision increases the likelihood that customers will find what they’re looking for quickly, reducing the time to purchase and boosting conversion rates.
2. Improved Customer Experience
A more personalized shopping experience leads to higher customer satisfaction. When users feel that the website “understands” their needs and preferences, they are more likely to engage with it, browse longer, and return in the future. For instance, if a returning customer sees product recommendations that align with their previous interests, they are more likely to explore those suggestions and make a purchase.
3. Higher Conversion Rates
Personalized search results directly contribute to higher conversion rates. By serving up relevant products, adaptive search reduces friction in the shopping journey, leading to faster decision-making and more purchases. The system can also recommend complementary products (cross-sells) and upgrades (up-sells) that match the user’s intent, further increasing the average order value.
4. Reduced Cart Abandonment
One of the main reasons for cart abandonment is the inability to find the right product or feeling overwhelmed by irrelevant search results. Adaptive search addresses this issue by streamlining the product discovery process and ensuring that the user is shown the most appropriate products right from the start, reducing the chances of abandoning the search or cart.
5. Scalability Across User Segments
Whether an e-commerce site serves millions of customers or a smaller, niche market, adaptive search is scalable and can provide personalized experiences for every user segment. It can adjust to the behavior of different customer types, ensuring that both casual browsers and power users receive tailored search results that meet their needs.
Real-World Applications of Adaptive Search in E-Commerce
Many leading e-commerce platforms are already leveraging adaptive search technology to enhance their customer experience. Here are a few examples:
- Amazon: Amazon’s search engine continuously learns from user interactions, offering personalized recommendations based on past purchases, browsing history, and frequently searched items. The adaptive nature of their system helps drive higher sales through tailored product recommendations.
- Zappos: Zappos uses adaptive search to suggest products that align with user preferences, such as showing specific brands or styles based on previous clicks and purchases.
- Sephora: Beauty retailer Sephora enhances its product discovery process through adaptive search by suggesting products based on customer preferences for certain brands, skin types, or makeup styles, all powered by machine learning algorithms.
Leveraging Adaptive Search for Personalized E-Commerce Experiences
As e-commerce continues to grow and competition intensifies, businesses need to adopt more sophisticated tools to stay ahead. Adaptive search offers a dynamic, personalized search experience that not only improves customer satisfaction but also drives business growth through higher conversion rates, reduced cart abandonment, and greater customer retention.
With the power of AI and machine learning, adaptive search systems are poised to revolutionize how shoppers find and purchase products online. As more e-commerce platforms integrate this technology, the future of search will be one where personalization and relevance take center stage, delivering a better, more intuitive shopping experience for users worldwide.