Predictive AI vs. Predictive Analytics: The Key Differences
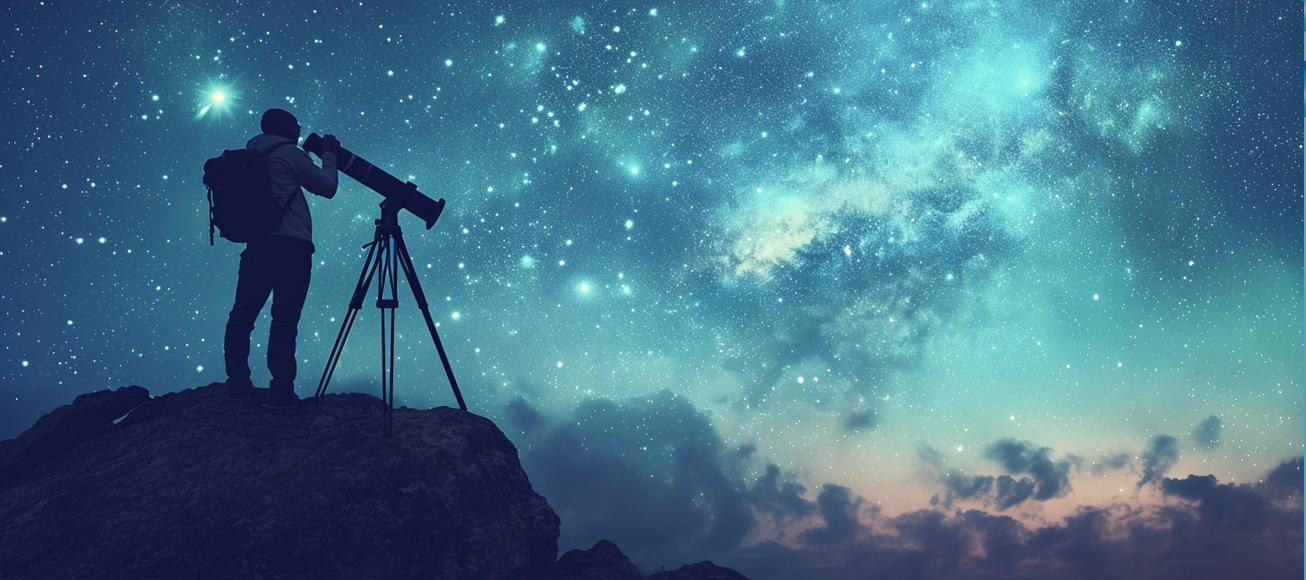
In a world where data drives decisions and customer expectations shift rapidly, predictive AI is transforming how organizations approach forecasting, personalization, and real-time engagement. By extending beyond the limits of traditional predictive analytics, predictive AI offers businesses a dynamic, self-learning capability to make accurate predictions and improve customer experiences. Here’s why predictive AI is increasingly critical for businesses aiming to thrive in a competitive landscape.
The Main Distinctions Between Predictive AI and Predictive Anaytics
While both predictive analytics and predictive AI are built to forecast outcomes, they differ in flexibility, accuracy, and adaptability. Traditional predictive analytics relies on historical data and statistical models to predict outcomes based on established patterns. Predictive AI, however, builds on this by integrating deep learning and continuous, self-adjusting algorithms, making it far more responsive to real-time changes in data and complex variables.
In short, while predictive analytics answers, “What will likely happen based on the past?”, predictive AI continuously adjusts its models to answer, “What is most likely to happen next, given the current context and new data?” The difference is substantial, especially in fast-paced, data-rich environments where real-time adjustments offer a competitive edge.
Feature | Predictive AI | Predictive Analytics |
Adaptability | Continuously self-optimizes and learns | Limited to static models |
Real-Time Processing | Incorporates real-time and dynamic inputs | Primarily historical data |
Complexity of Models | Deep learning, neural networks, and advanced machine learning | Statistical and basic machine learning |
Data Types | Structured and unstructured data, including text and audio | Structured, quantitative data |
Human Intervention | Minimal human input needed, adaptive learning | Requires human recalibration |
Accuracy Over Time | Constantly improves accuracy with continuous data input | Accuracy can degrade if not updated |
Key Benefits of Predictive AI Over Traditional Predictive Analytics
- Real-Time Adaptability and Responsiveness
Predictive AI operates by processing and analyzing real-time data alongside historical information. This real-time adaptability allows businesses to stay relevant and responsive to current conditions without manual recalibration. For example, a traditional predictive analytics model might recommend the same strategy until manually updated, but predictive AI can automatically adjust its recommendations based on the latest inputs, keeping forecasts accurate even as trends shift. - Continuous Self-Learning
A core component of predictive AI is its ability to learn autonomously. Unlike predictive analytics models, which must be periodically updated to remain accurate, predictive AI models learn and evolve with each new data input. This continuous self-learning not only enhances accuracy but also reduces the need for manual oversight. Over time, predictive AI’s accuracy improves, enabling it to identify and adapt to subtle changes in customer behavior, industry trends, and market shifts more effectively. - Enhanced Personalization and Relevance
Predictive AI is particularly beneficial in applications where customer engagement and personalization are key. By analyzing behavioral patterns, purchasing preferences, and demographic details in real time, predictive AI offers deeper insights that help tailor user experiences to each individual. From e-commerce recommendations to personalized content delivery, predictive AI enables businesses to dynamically adapt offerings to each customer’s unique needs, boosting engagement and satisfaction. - Scalability for Complex Applications
Predictive AI’s advanced architecture makes it well-suited for large-scale, complex applications. Traditional predictive analytics models are constrained by their capacity to process only structured, historical data, making them less ideal for environments where unstructured data (like social media posts or customer reviews) is prevalent. Predictive AI, on the other hand, can handle vast volumes of diverse data types—including images, text, and audio—scaling effectively with business growth and data complexity. - Improved Decision-Making
Predictive AI enables businesses to make more informed, data-driven decisions quickly. By delivering high-accuracy predictions grounded in both historical and real-time data, predictive AI allows managers to act on insights confidently. Whether it’s predicting customer churn, optimizing inventory, or detecting fraud, predictive AI provides a level of granularity that enhances decision-making and allows for better risk management.
The Challenges of Relying Solely on Predictive Analytics
While predictive analytics has long been valuable for businesses, its limitations become apparent as data environments grow more complex. Traditional predictive models lack the adaptability needed to respond to rapid changes in real-time, which can lead to outdated or even inaccurate predictions if not regularly updated.
Limited Adaptability
Predictive analytics relies on static algorithms, which are typically built based on historical patterns and cannot update autonomously. As a result, these models often fall short when applied to dynamic environments where data evolves quickly, such as e-commerce, finance, and customer service. In contrast, predictive AI’s adaptive nature allows it to stay aligned with current conditions, ensuring accuracy even as data changes.
Dependency on Human Intervention
Traditional predictive analytics requires periodic updates, recalibration, and monitoring, often demanding the attention of data scientists or analysts. In fast-paced environments, this reliance on human intervention can become a bottleneck, preventing businesses from responding quickly to opportunities or risks. Predictive AI’s self-learning capabilities reduce the need for manual oversight, enabling a more streamlined, agile approach to data intelligence.
Choosing Predictive AI: Strategic Advantages for Businesses
When considering an upgrade from predictive analytics to predictive AI, businesses should consider the following strategic advantages that predictive AI can bring to their operations and customer engagement strategies:
Competitive Differentiation
As AI-driven tools become more accessible, companies across industries are rapidly adopting predictive AI to gain a competitive edge. Businesses that implement predictive AI can offer more personalized, adaptive experiences, enhancing customer satisfaction and loyalty. By staying agile and responsive, predictive AI-powered companies differentiate themselves in crowded markets, delivering value in a way that traditional analytics cannot match.
Improved Operational Efficiency
Predictive AI’s self-learning algorithms and real-time adaptability streamline operations, reduce the need for constant model updates, and free up time for teams to focus on high-value tasks. This improved efficiency translates into significant cost savings, especially in large organizations where managing and updating traditional predictive models can be resource-intensive.
Enhanced Customer Retention and Engagement
Predictive AI provides insights that allow for highly personalized interactions with customers, which is essential for fostering loyalty and engagement. From hyper-relevant product recommendations to personalized support experiences, predictive AI ensures customers receive timely, meaningful interactions that enhance satisfaction and drive retention.
Scalability for Evolving Data Landscapes
The data environment is growing exponentially, with businesses generating more data than ever before. Predictive AI’s ability to scale with data complexity and volume enables businesses to keep pace with this growth. Traditional predictive analytics, limited in its handling of complex data types, often struggles to keep up, while predictive AI thrives in dynamic, data-rich settings.
Factors to Consider When Adopting Predictive AI
While predictive AI offers substantial advantages, implementing it effectively requires a solid foundation and thoughtful planning. Businesses should consider the following factors when adopting predictive AI:
Data Infrastructure and Quality
Predictive AI relies on high-quality, comprehensive datasets to deliver accurate predictions. Organizations considering predictive AI should ensure their data infrastructure is equipped to handle large volumes of data in real-time. Clean, relevant data is essential, as predictive AI algorithms learn from each input, and poor data quality could impair performance.
Expertise and Training
Predictive AI is highly effective but requires a degree of technical expertise to implement and manage. Many businesses find that partnerships with AI technology providers or investments in skilled data science teams can ease the transition. Additionally, training team members to understand the insights generated by AI can help ensure these insights are effectively incorporated into business strategies.
Regulatory Compliance
Predictive AI must adhere to data privacy and regulatory standards, especially in industries like finance and healthcare. Businesses should ensure that any predictive AI implementation complies with relevant regulations, such as GDPR, CCPA, or industry-specific guidelines, and prioritize transparent data practices to maintain trust.
Future Trends in Predictive AI
Predictive AI is evolving quickly, and future advancements will further enhance its capabilities. As technology advances, predictive AI models will become even more accurate, autonomous, and easy to integrate with existing business systems. Key trends on the horizon include:
- Explainable AI: Making AI-driven insights more transparent will allow business users to better understand and trust predictions, addressing one of the main challenges with current predictive AI models.
- Augmented Predictive AI: Integrating predictive AI with other emerging technologies, such as the Internet of Things (IoT) and blockchain, will unlock new applications and efficiencies across sectors.
- Increased Accessibility: As predictive AI platforms become more user-friendly and cost-effective, more organizations will be able to leverage this technology without needing a large in-house data science team.
Unlocking Strategic Value with Predictive AI
For businesses aiming to stay competitive, responsive, and data-driven, predictive AI is a transformative solution that surpasses traditional predictive analytics in adaptability, accuracy, and scalability. By choosing predictive AI, organizations can unlock new levels of insight, enhance customer experiences, and streamline operations, all while staying agile in an ever-changing business environment. While implementing predictive AI requires careful planning, the benefits it brings in terms of efficiency, customer retention, and decision-making make it an essential tool for modern businesses.